ML-based, Data-driven Infrastructure to Accelerate the Modeling and Simulation of Nanostructures
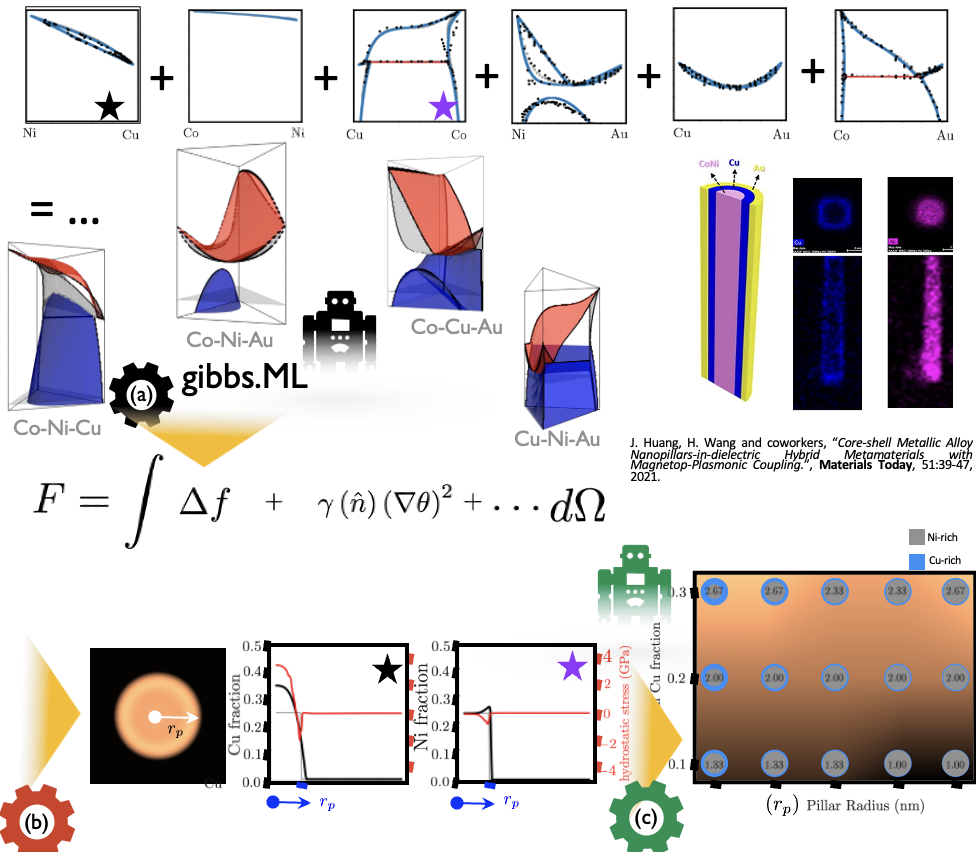
We present a data-driven numerical framework that allows us to integrate physics-based machine-learning (ML) based strategies to rapidly incorporate multicomponent, multiphysicalfunctionalities of advanced metamaterials. The developed ML strategy incorporates complementary binary phase diagram data into ternaries, quaternaries, etc., descriptions that can be readily used to describe the local equilibrium in multicomponent nanopillars for photonics applications.
This works defines a numerical platform to infer nanostructures of tailored functionality for advanced integrated circuits.