DMREF Specific Highlights
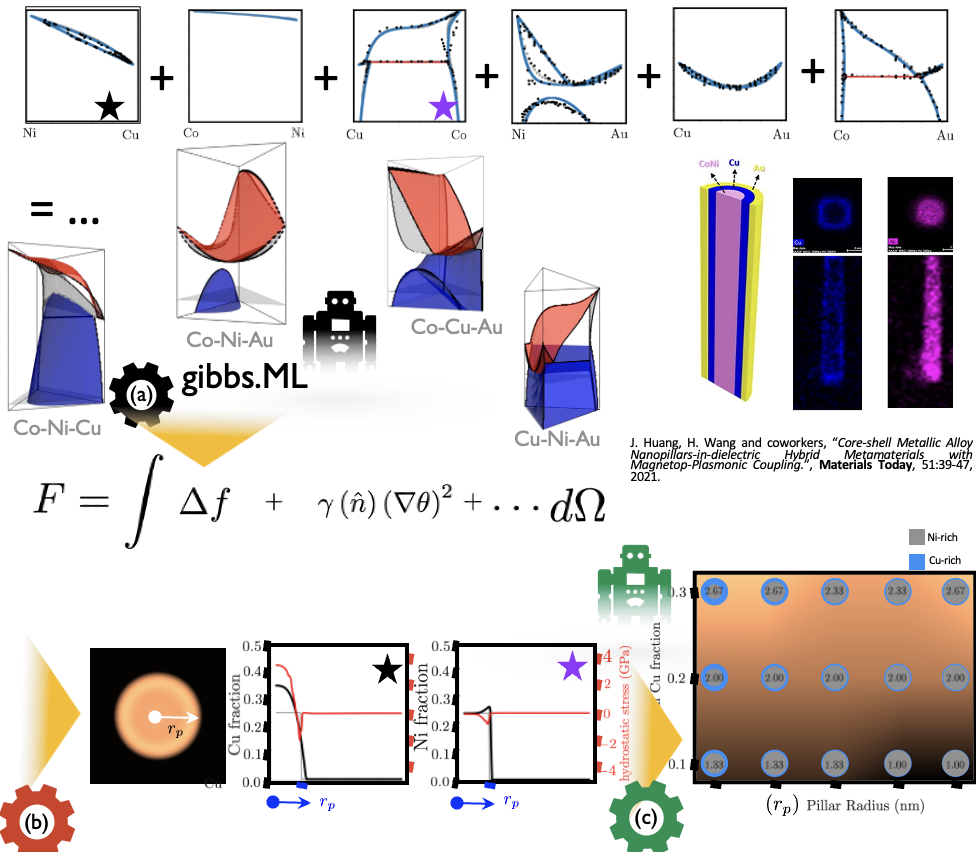
ML-based, Data-driven Infrastructure to Accelerate the Modeling and Simulation of Nanostructures
6/24/2024 | Haiyan Wang (Purdue University)
ML-powered data analytics framework, incorporating the effect available experimental thermodynamic data into phase field models as generated by gibbs.ML in quaternary Ni-Cu-Co-Au-based nanopillars. The generated model allows to streamline the simulation of the segregation dynamics during processing, and generate maps as a function of composition and morphology.
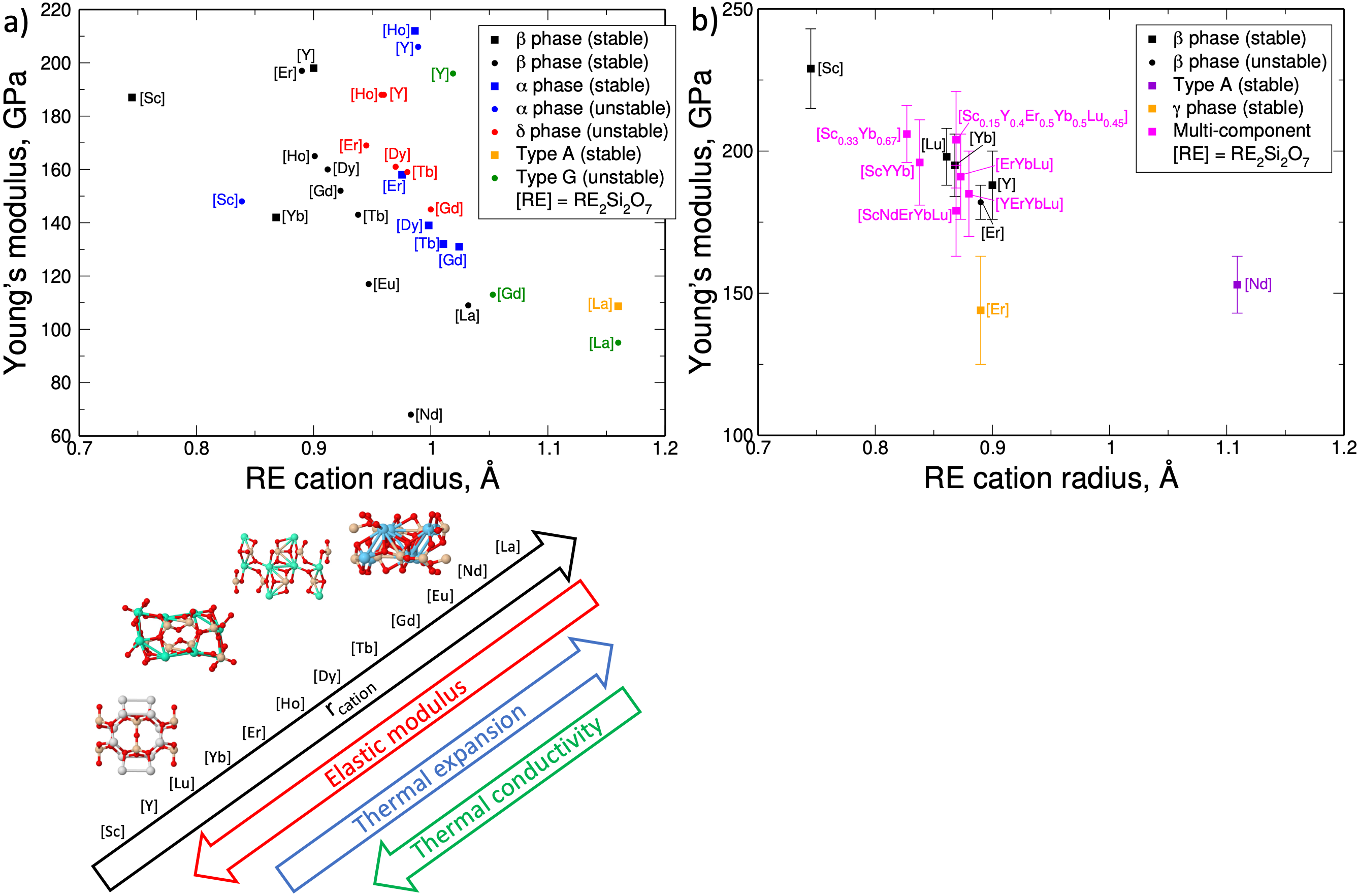
Design rules for the thermal and elastic properties of rare-earth disilicates
6/5/2024 | Elizabeth Opila – University of Virginia
Computational and experimental data were combined to develop design rules for rare-earth disilicates to enable tuning of thermo-mechanical properties to better match the SiC substrate. In the case of Young’s modulus and thermal expansion, these trends also hold for multi-component systems; while the thermal conductivity of multi-component systems is noticeably lower, indicating the potential of such materials to also act as thermal barriers.
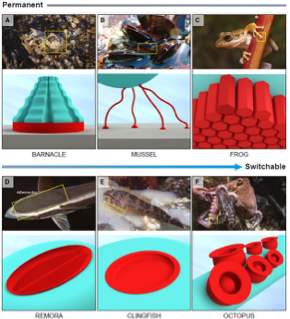
Bioinspired Materials for Underwater Adhesion with Pathways to Switchability
5/30/2024 | M. Bartlett (Virginia Tech.), B. Lee (MI Tech.) R. Long (U. CO-Boulder), G. Gu (U. CA-Berkeley)
Strong adherence to underwater or wet surfaces for applications like tissue adhesion and underwater robotics is a significant challenge. This is especially apparent when switchable adhesion is required that demands rapid attachment, high adhesive capacity, and easy release. Nature displays a spectrum of permanent to reversible attachment from organisms ranging from the mussel to the octopus, providing inspiration for underwater adhesion design that has yet to be fully leveraged in synthetic systems. Here, we review the challenges and opportunities for creating underwater adhesives with a pathway to switchability.
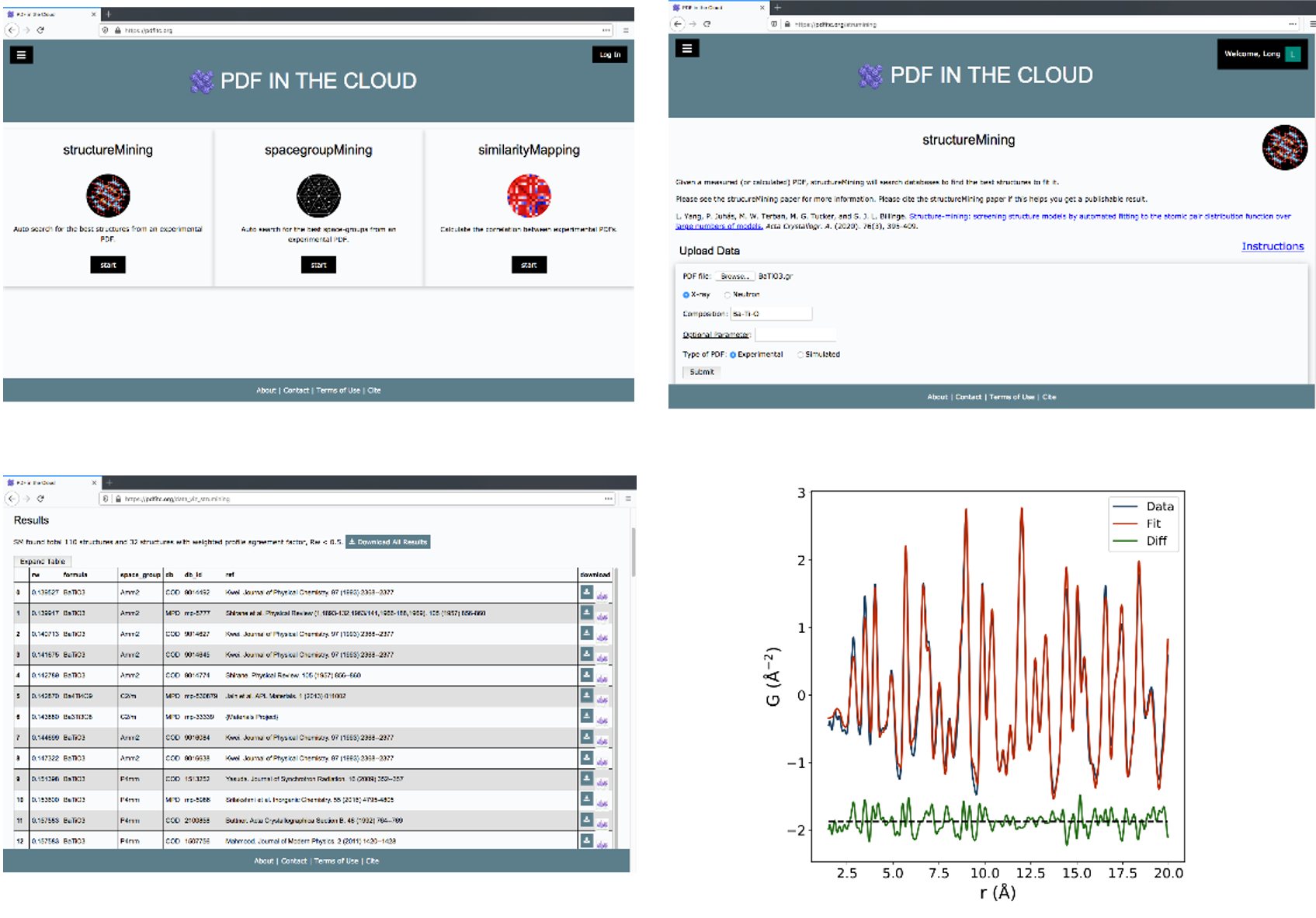
An AI-driven, Cloud-based, Materials Discovery Platform for Nanomaterial Structure: PDFitc
5/28/2024 | S. Billinge, Q. Du, D. Hsu (Columbia U.)
Discovery of novel functional materials relies on a quantitative understanding of nanostructure. There is a need for advanced artificial intelligence (AI) and machine learning (ML) approaches to nanostructure determination
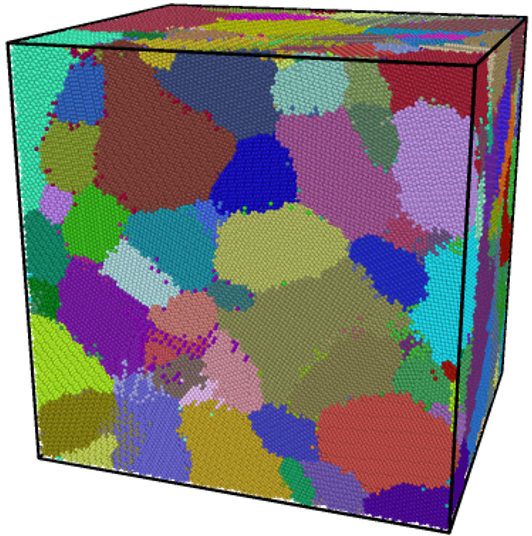
Data Flow Between Experiment, Continuum Models, and Atomistic Models
5/22/2024 | Gregory S. Rohrer, Carnegie Mellon University
A key challenge of learning from the outcomes of experiments and simulations is consistency of data formats and analysis techniques. We have developed the ability to seamlessly integrate findings from atomistic simulations, mesoscale simulations, and experiments.
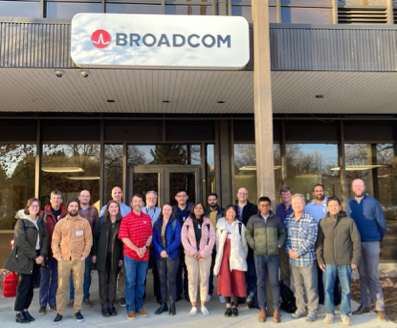
Advancing the State of the Art In Piezoelectric Materials through Industry Partnership
5/14/2024 | David Martin (Broadcom)
As a GOALI project, the relationship between the DMREF team led by Geoff Brennecka and industry partner, Broadcom, is key to the success of the project. Broadcom is a global leader in Thin-film Bulk Acoustic Resonator (FBAR) products and a major supplier of radio frequency (RF) filters to leading smartphone manufacturers.
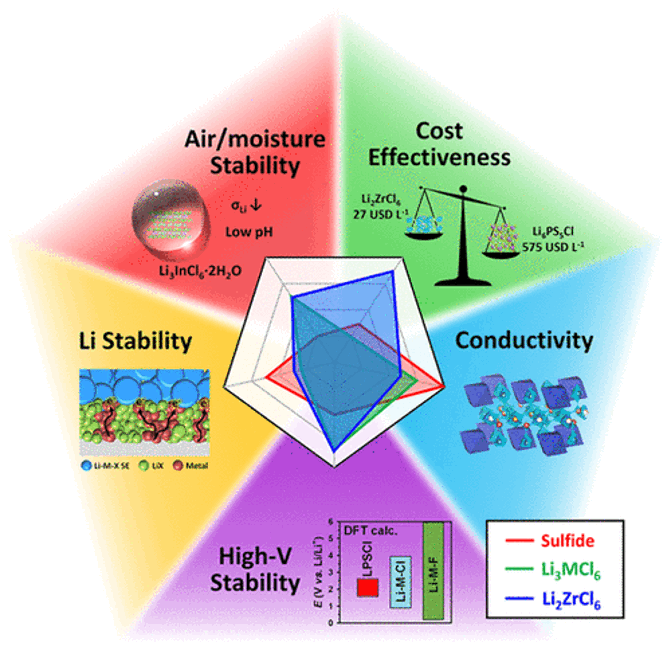
Review: Emerging Halide Superionic Conductors for All-Solid-State Batteries: Design, Synthesis, and Application
5/10/2024 | Yifei Mo (University of Maryland)
Recently, halide superionic conductors have emerged as promising solid electrolyte (SE) materials for all-solid-state batteries (ASSBs), owing to their inherent properties combining high Li+ conductivity, good chemical and electrochemical oxidation stabilities, and mechanical deformability, compared to sulfide or oxide SEs. In this Review, recent advances in halide Li+- and Na+-conducting SEs are comprehensively summarized.
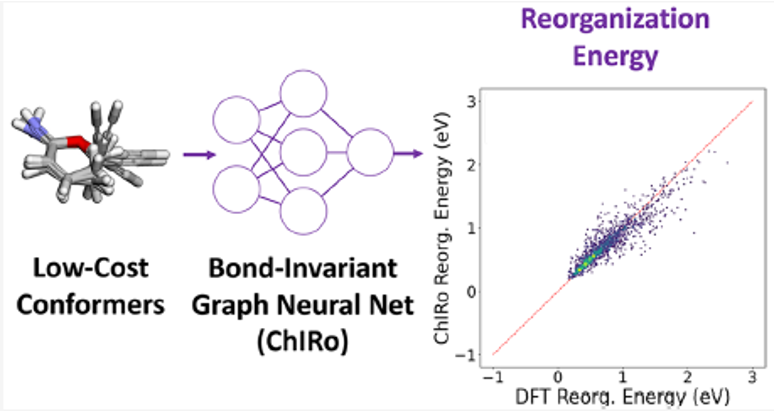
Reorganization Energy Predictions with Graph Neural Networks
4/22/2024 | Daniel Tabor
These results demonstrate the feasibility of reorganization energy predictions on the benchmark QM9 data set without needing DFT-optimized geometries and demonstrate the types of features needed for robust models that work on diverse chemical spaces.
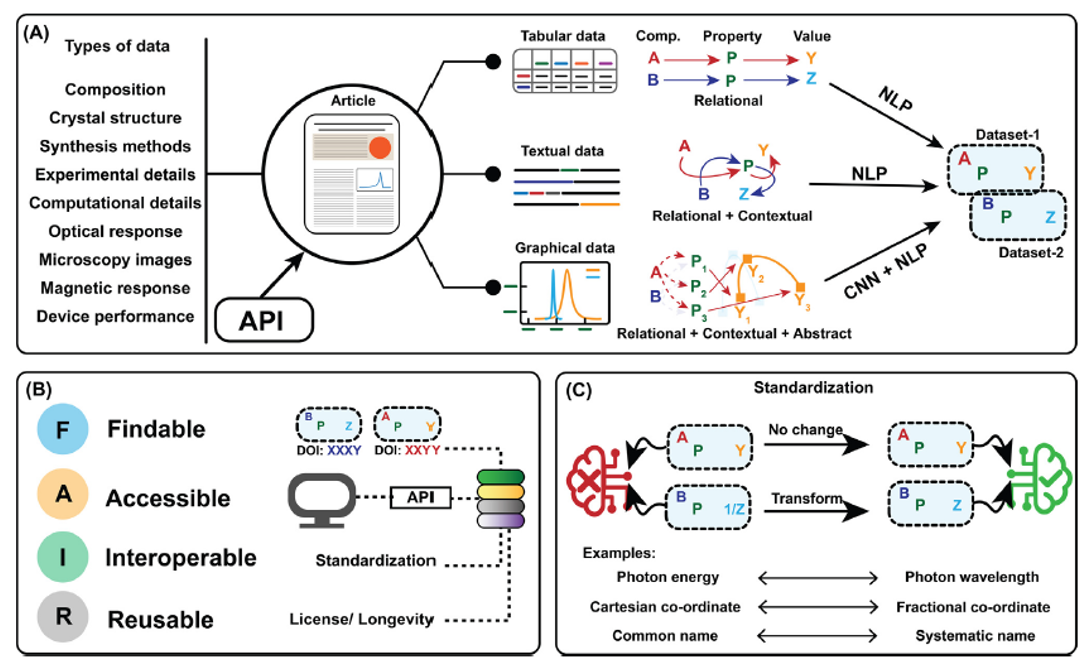
Curated Materials Data of Hybrid Perovskites
10/1/2023 | R. Chakraborty and V. Blum
Hybrid perovskites have emerged as a group of semiconductors that can solve key problems involving efficiency and production in optoelectronics and spintronics research. Over the past decade, this field has evolved to a point where the literature contains an enormous volume of chemical and physical information. The dispersed nature of the large, rapidly growing body of hybrid perovskite materials data poses a barrier to systematic discovery efforts, which can be solved by materials property databases, either by high-throughput or by systematic, accurate human-curated efforts.
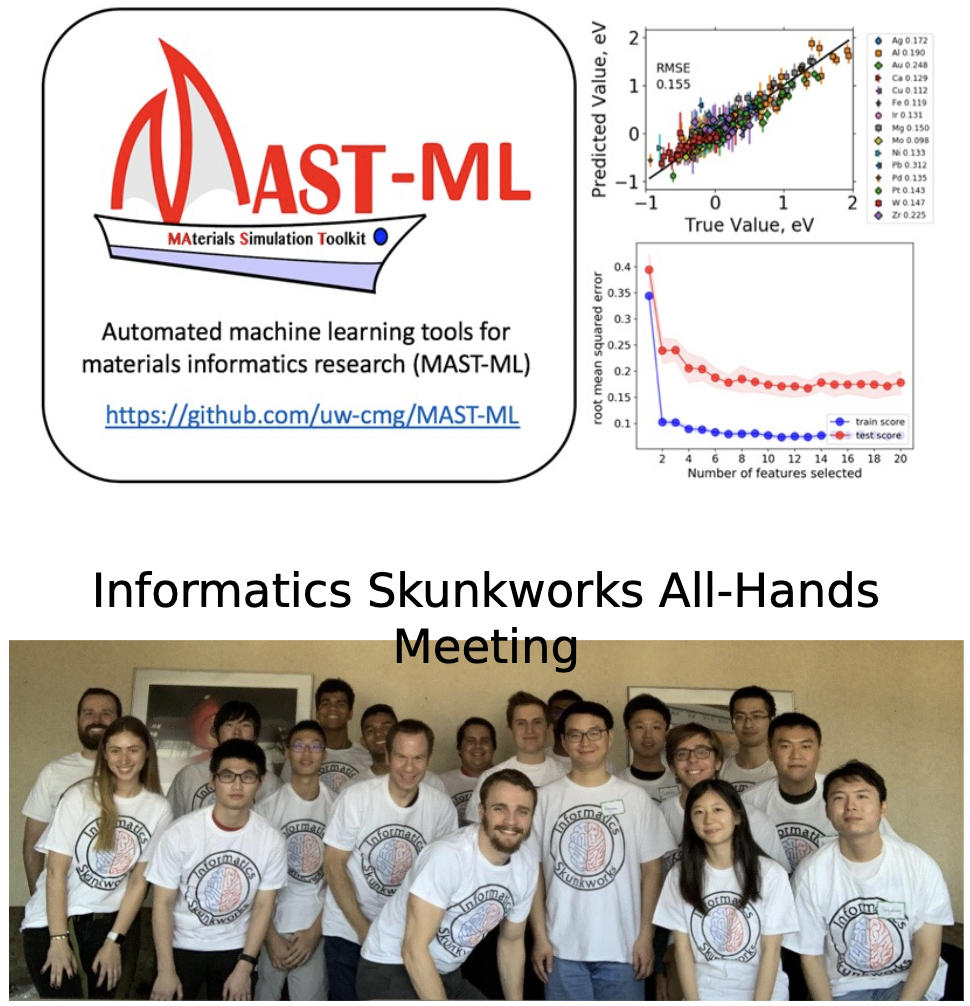
Materials Simulation Toolkit
3/10/2023 | Dane Morgan (University of Wisconsin)
Showing 1 to 10 of 31