Machine Learning Accelerated First-principles Study of the Hydrodeoxygenation of Propanoic Acid
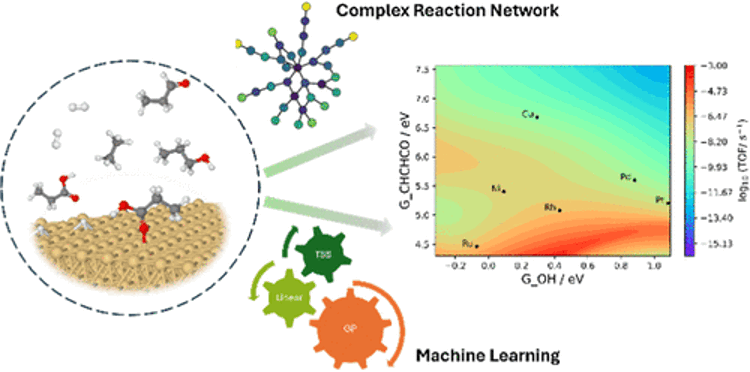
The study of the usage of biomass as an alternative to fossil fuels has greatly increased due to environmental and climate issues brought about by the combustion of fossil fuels and theirderivatives. However, biomass-derived fuels have their drawbacks, such as high viscosity, poor oxidation stability, low energy density, and high cloud point temperature, due to their high oxygen content. Deoxygenation therefore becomes very important in the chemical utilization of biomass. Currently, the heterogeneous catalyzed hydrodeoxygenation (HDO) process, is widely used for the conversion of biomass to valuable products. Conventional petrochemical-based HDO catalysts, such as sulfided NiMo/Al2O3 and CoMo/Al2O3, cannot be used for the conversion of biomass due to the low sulfur content in biomass, high sulfur content in the final product, and short catalyst lifetimes. To overcome these limitations of conventional sulfidedcatalysts, new metal catalysts must be developed for the HDO of biomass, which requires a comprehensive understanding of the mechanism of HDO on the catalyst surface. The complex reaction network of catalytic biomass conversions often involves hundreds of surface intermediates and thousands of reaction steps, greatly hindering the rational design of metal catalysts for these conversions. Here, a framework of machine learning (ML)-accelerated first principles studies is presented for the HDO of propanoic acid over transition metal surfaces. The microkinetic model (MKM) was initially parametrized by ML-predicted energies and iteratively improved by identifying the rate-determining species and steps, computing their energies by density functional theory (DFT), and re-parameterizing the MKM until all the RDS are computed by DFT. It was found that a high accuracy in adsorption free energies is more important for a reliable MKM than a high accuracy in transition state free energies.