An AI-driven Workflow for Accelerated Morphology, Electronic Structure, and Phase Characterization
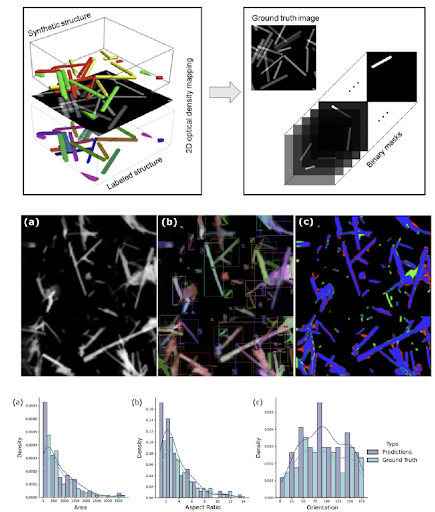
We have devised a workflow for deep learning of feature dimensions across electron microscopy, X-ray microscopy, and X-ray ptychography data. The workflow is based on a Mask R-CNN architecture and enables segmentation of different nanoparticle morphologies and determination of particle dimensions, size distributions, orientation, and aspect ratio. The workflow comprises the following elements: (a) Feature extraction; (b) region of interest alignment; and (c) mask and bounding box prediction and classification.
Despite substantial variations in spatial resolution, particle dispersion densities, and image contrast mechanisms across different imaging tools, the model scores well across all the considered microscopy data thus demonstrating the versatility of the neural network in segmentation tasks. This work has now been published in npj Computational Materials.
A web-based interactive tool for on-the-fly image analysis has been made available on Github: https://linbinbin92-v2o5-app-v2o5-app-6l2ksq.streamlit.app
Additional Materials
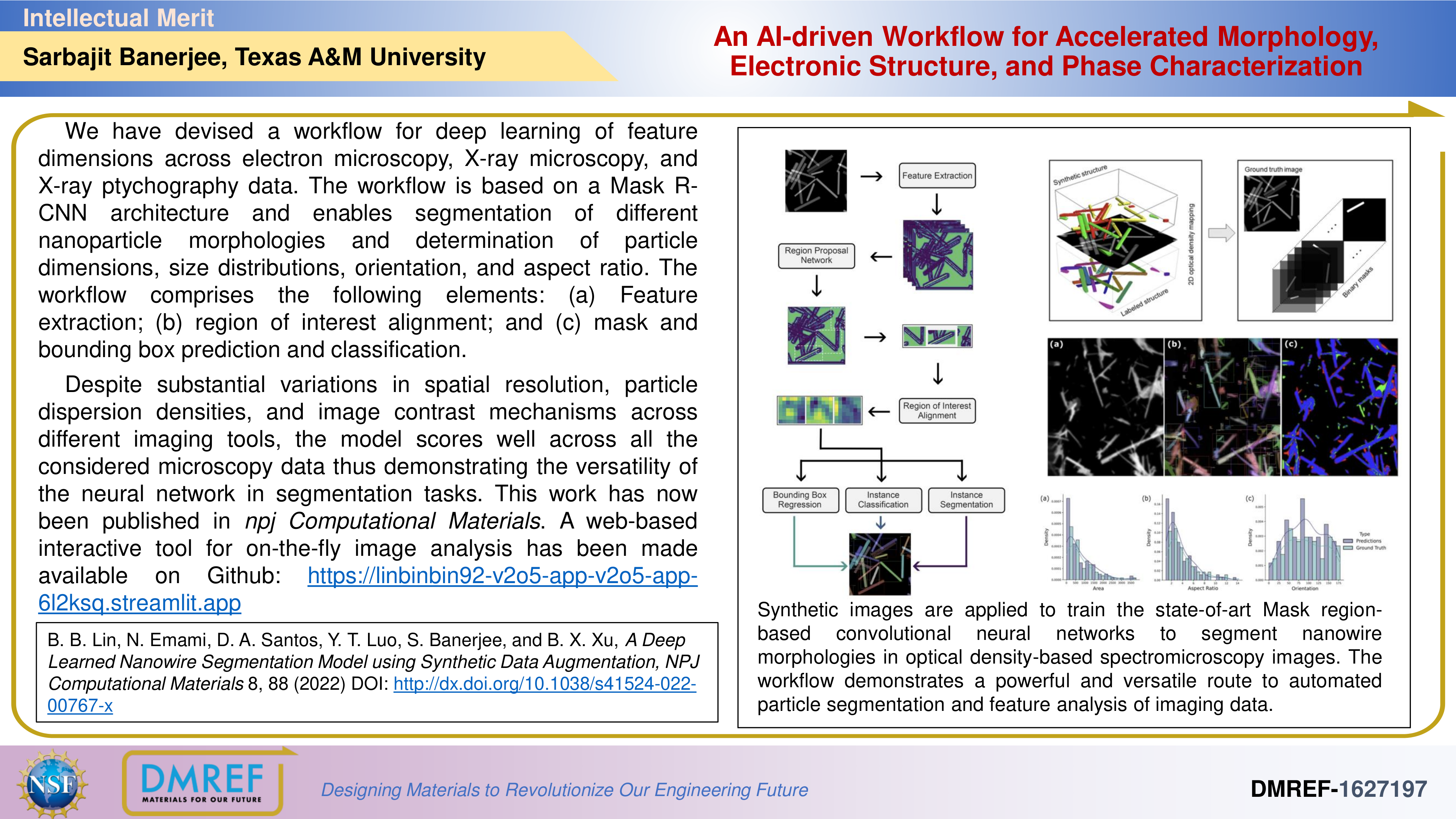