Computationally Driven Genetically Engineered Materials
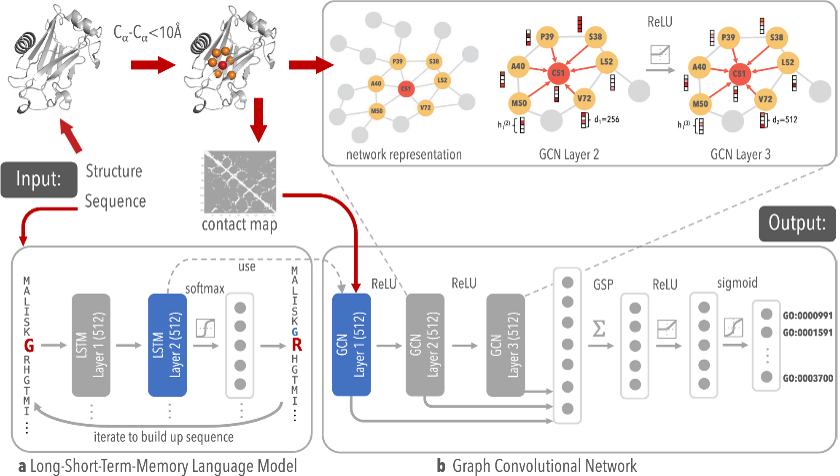
Development of protein biomaterials that are capable of self-assembly into hydrogels has potential in biomedical applications including drug delivery and tissue engineering. A two-stage architecture, called DeepFRI, has been recently developed where functional salinity is established by training its algorithm on annotated structures from PDB and SWISS-MODEL and applying weighted class activation mapping of residues that are critical to function (Fig. 1). Similarly, a two-stage architecture has been developed to help understand the driving mechanism for gelation of coiled-coil proteins that will enable the design of new materials with desired phase-behavior. To this end, we have studied the conditional properties of self-assembly of Q using high-throughput micro-rheology and begun developing not only improved variants of Q, but a machine learning algorithm program that will quantify the metrics that drive self-assembly and provide optimum sequence design for improved gelation and material properties for a coiled-coil. Results of this work will provide insight into the driving mechanisms for new polymeric materials and provide a platform for their design. From a database of coiled-coil sequences derived from a randomized Monte-Carlo search algorithm, sequence will be matched to electrostatic potential which will be used to map the time to gelation using a subset of variants that fill the compositional space of electrostatic patches (Fig. 2 highlights the qualitative results thus far using classifiers gel and no gel).