The Synthesis Genome: Data Mining for Synthesis of New Materials
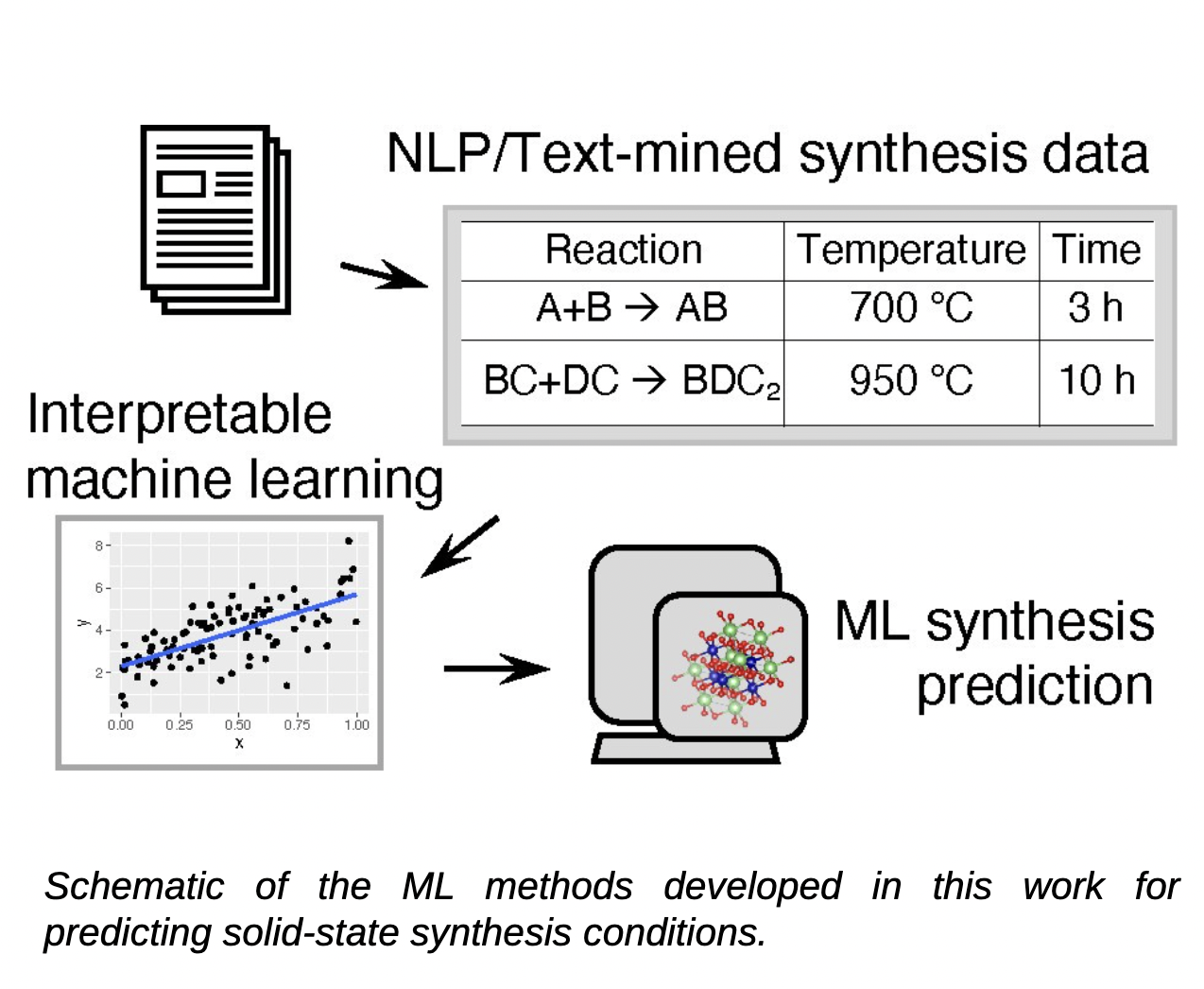
Interpretable machine-learning (ML) models were developed to predict two key solid-state synthesis conditions that must be specified for any reaction: heating temperature and heating time.
Our ML results achieve a mean absolute error (MAE) of 140 °C ∼for heating temperature prediction. The predicted synthesis time is typically within a factor of 2 of the reported value.
Heating temperature prediction is dominated by precursor properties, which we hypothesize to be linked to reaction kinetics. Heating time prediction is dominated by experimental operations, which may be indicative of human selection bias.
Moreover, our model formally proves that Tamman’s rule, which was firstly applied to alloys, can be extended to ionic compounds as well.
Additional Materials
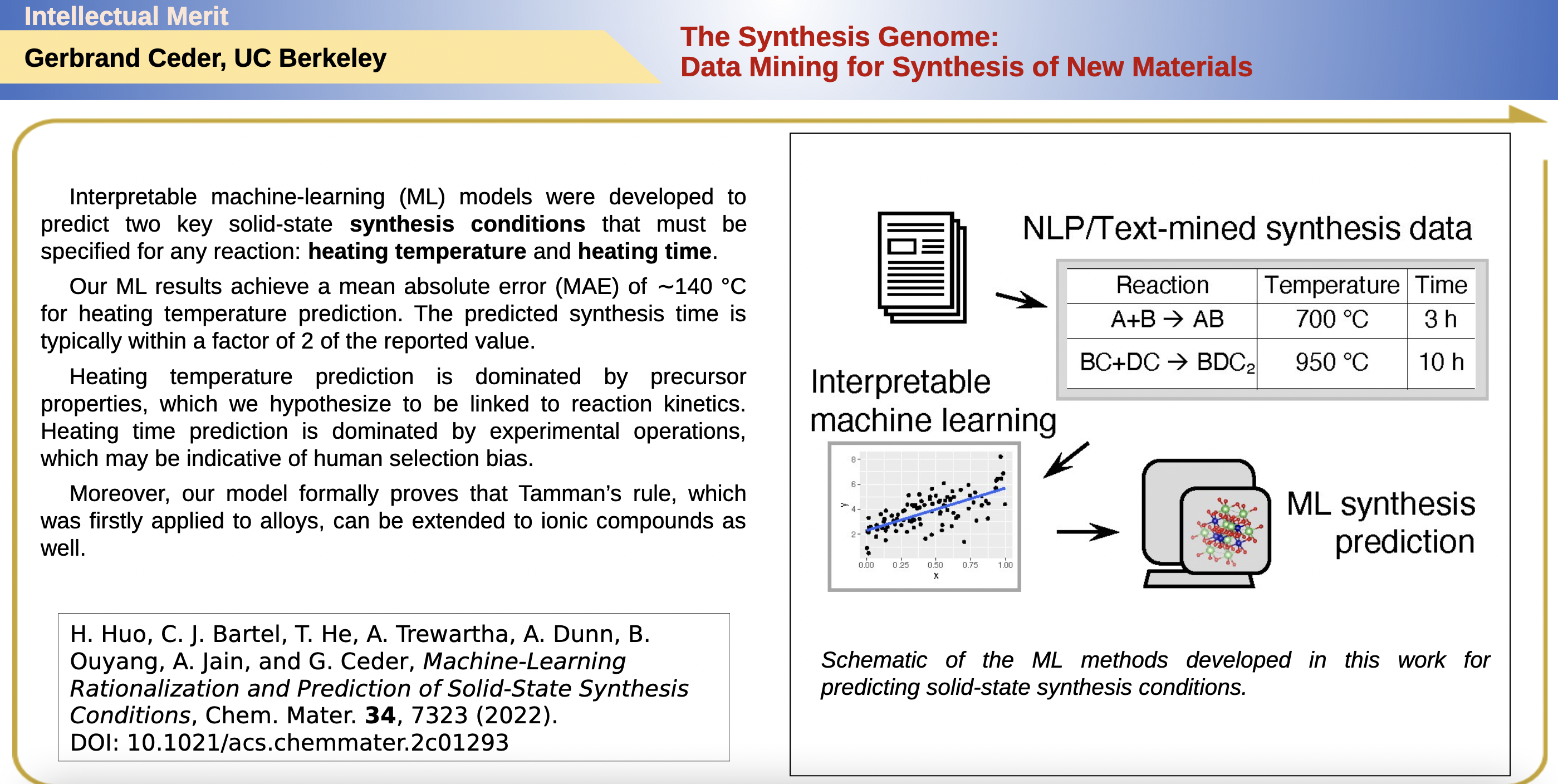