Designing Exceptional Gas-separation Membranes with Machine Learning
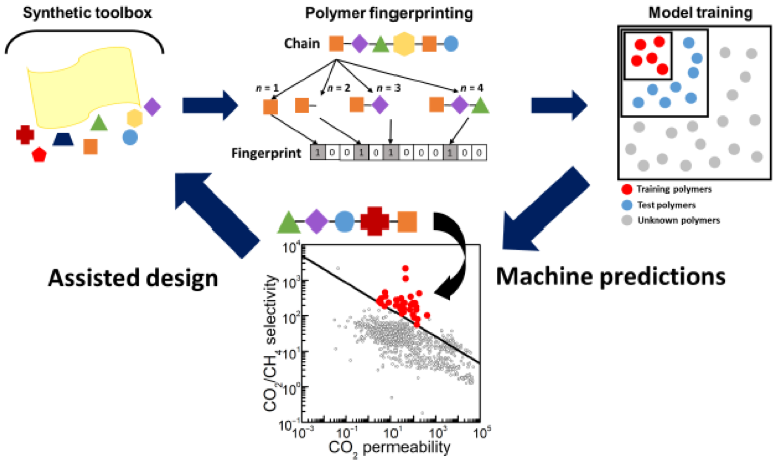
Polymer membranes are used for a variety of gas separations including the removal of carbon dioxide from natural gas, oxygen from air, hydrogen recovery, and carbon capture. The field of polymer membrane design is primarily based on empirical observation, which limits discovery of new materials optimized for separating a given gas pair.
Instead of relying on exhaustive experimental investigations, this team has trained a machine learning algorithm, through use of a topological, path-based hash of the polymer repeating unit. Gas permeation data from about 750 polymers were used for each gas to predict behavior of about 11,000 polymers. To test the algorithm’s accuracy, they synthesized two of the most promising polymer membranes predicted by this approach and found that they exceeded the upper bound for CO2/CH4 separation performance.
Instead of relying on exhaustive experimental investigations, this team has trained a machine learning algorithm, through use of a topological, path-based hash of the polymer repeating unit. Gas permeation data from about 750 polymers were used for each gas to predict behavior of about 11,000 polymers. To test the algorithm’s accuracy, they synthesized two of the most promising polymer membranes predicted by this approach and found that they exceeded the upper bound for CO2/CH4 separation performance.
Related Project: Designing Optimal Nanoparticle Shapes and Ligand Parameters for Polymer-Grafted Nanoparticle Membranes
Additional Materials
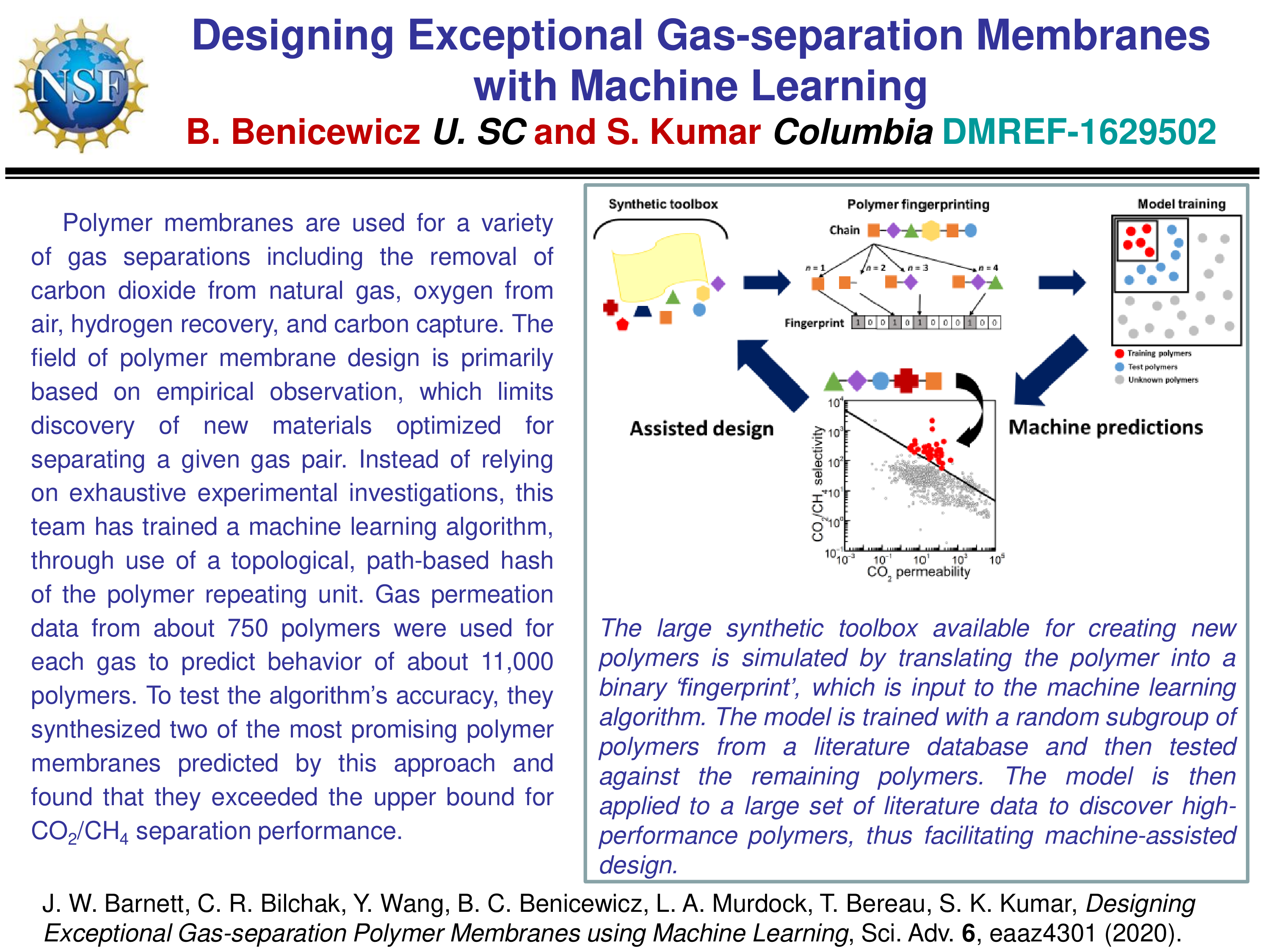