Data-driven Shape Memory Alloy Discovery using Artificial Intelligence Materials Selection (AIMS) Framework
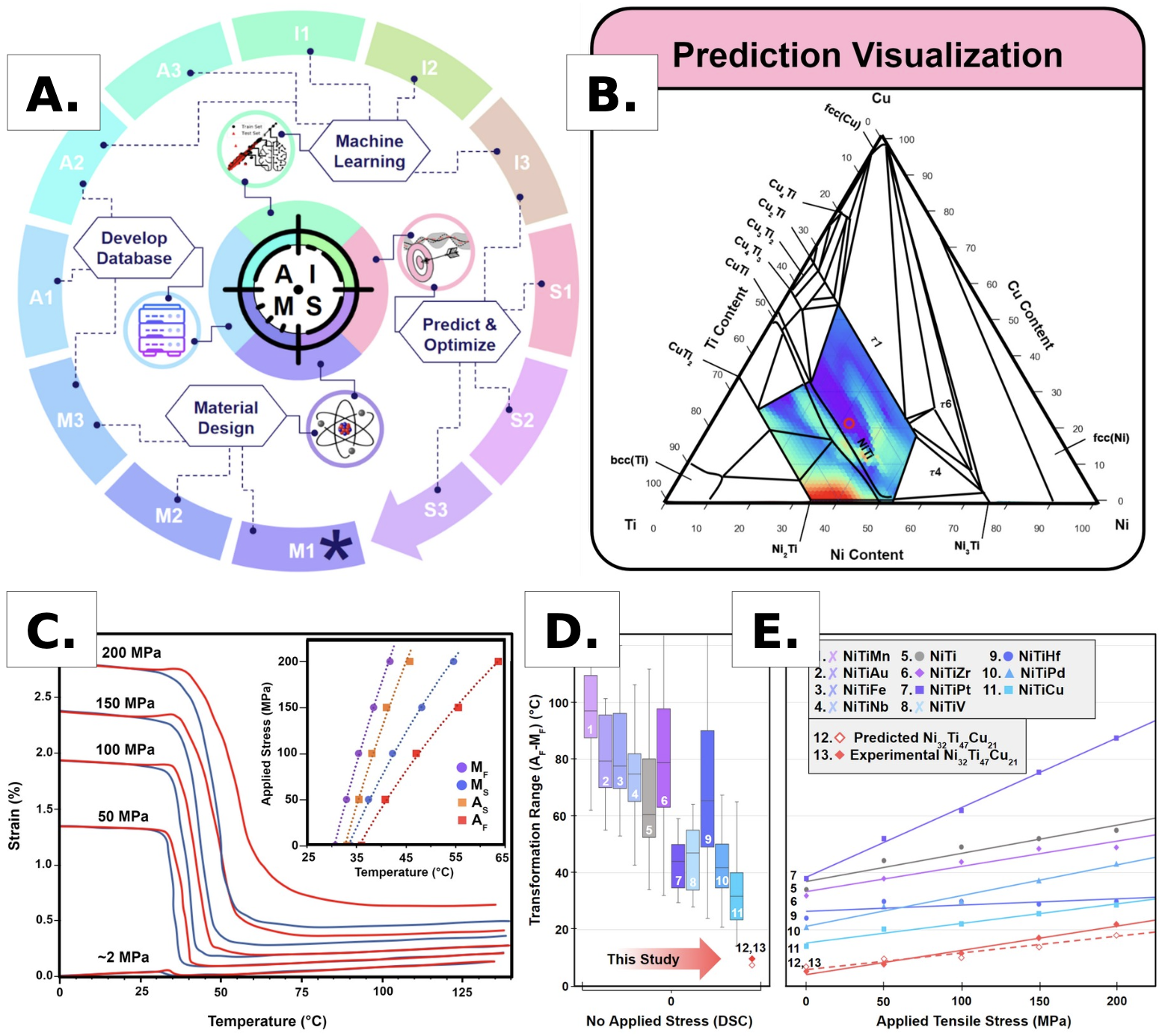
Problems Associated with Shape Memory Alloys (SMA)
• Thermal hysteresis – the difference in temperature for the forward versus reverse phase transformation – limits application.
• Consequences of thermal hysteresis are a larger required temperature excursion for application and reduced efficiency.
• Previous studies have focused on minimizing hysteresis under no stress, but not under applied stress.
New SMA Discovery via Machine Learning
New SMA Discovery via Machine Learning
• Materials informatics framework (A) was developed and used to analyze a SMA dataset of ~6,000 experimental data entries, constructed from raw data available.
• Ni32Ti47Cu21 (B, see red dot)was predicted by deep neural network regressors to have a small thermal hysteresis under stress.
• Ni32Ti47Cu21 has the smallest thermal hysteresis reported in the literature, based on experimental measurements (C) and comparison to literature under zero stress (D) and applied stress (E).
Additional Materials
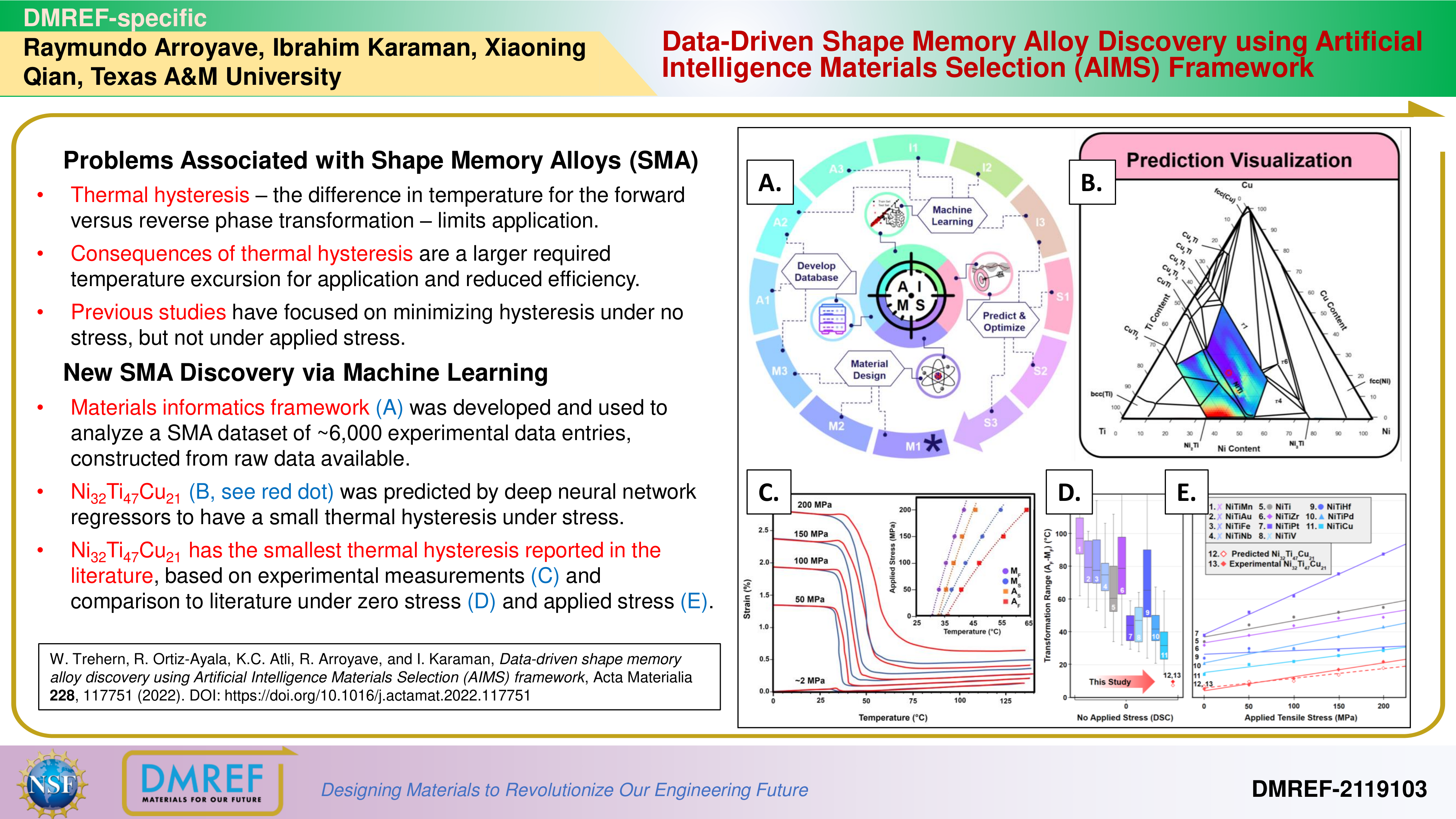