ML-based, Data-driven Infrastructure to Accelerate the Modeling and Simulation of Nanostructures
ML-powered data analytics framework, incorporating the effect available experimental thermodynamic data into phase field models as generated by gibbs.ML in quaternary Ni-Cu-Co-Au-based nanopillars. The generated model allows to streamline the simulation of the segregation dynamics during processing, and generate maps as a function of composition and morphology.
Haiyan Wang (Purdue University)
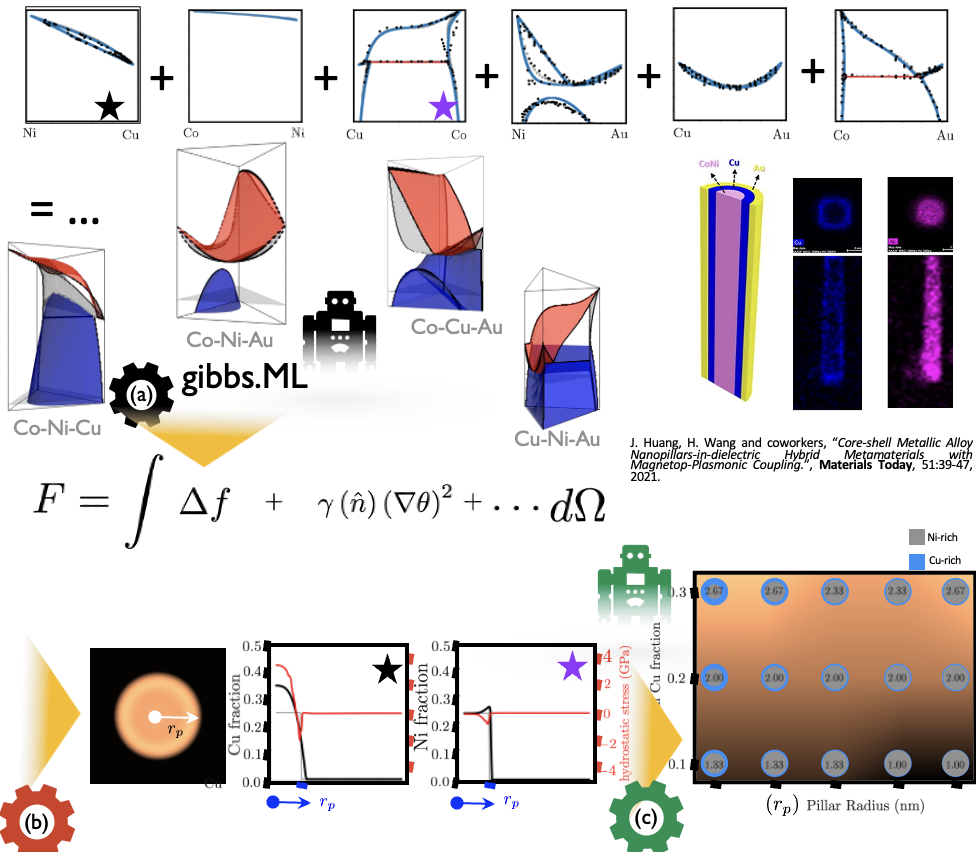
We present a data-driven numerical framework that allows us to integrate physics-based machine-learning (ML) based strategies to rapidly incorporate multicomponent, multiphysicalfunctionalities of advanced metamaterials. The developed ML strategy incorporates complementary binary phase diagram data into ternaries, quaternaries, etc., descriptions that can be readily used to describe the local equilibrium in multicomponent nanopillars for photonics applications.
This works defines a numerical platform to infer nanostructures of tailored functionality for advanced integrated circuits.