Data Centric Nanocomposite Design via Mixed-variable Bayesian Optimization
With an unprecedented combination of mechanical and electrical properties, polymer nanocomposites have the potential to be widely used across multiple industries. Tailoring nanocomposites to meet application specific requirements remains a challenging task, owing to the vast, mixed-variable design space that includes composition and microstructures of the nanocomposite material.
L. C. Brinson (Duke U.), W. Chen (Northwestern U.), L. Schadler (U. VT)
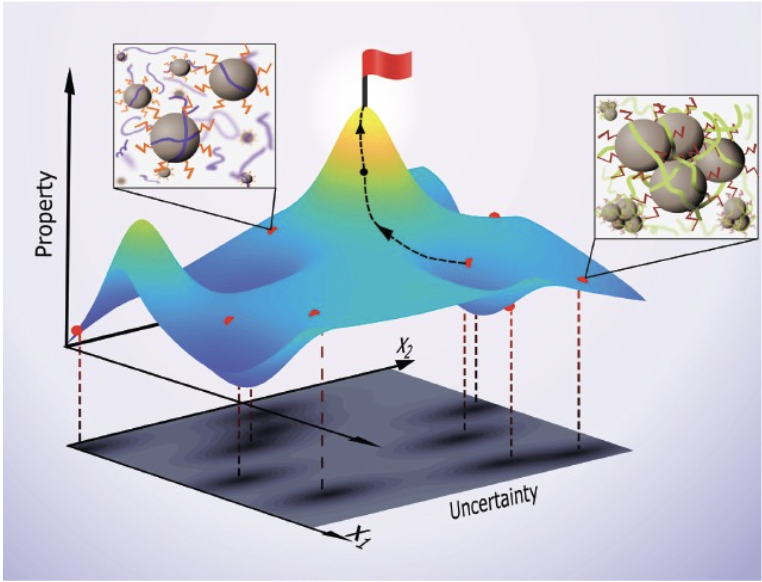
With an unprecedented combination of mechanical and electrical properties, polymer nanocomposites have the potential to be widely used across multiple industries. Tailoring nanocomposites to meet application specific requirements remains a challenging task, owing to the vast, mixed-variable design space that includes composition and microstructures of the nanocomposite material. Modeling properties of the interphase, the region surrounding a nanoparticle, introduces additional complexity to the design process and requires computationally expensive simulations. As a result, previous attempts at designing polymer nanocomposites have focused on finding the optimal microstructure for only a fixed combination of constituents. Here, a data centric design framework is proposed to concurrently identify optimal composition and microstructure using mixed-variable Bayesian optimization. This framework integrates experimental data with state-of-the-art techniques in interphase modeling, microstructure characterization and reconstructions and machine learning. Latent variable Gaussian processes (LVGPs) quantifies the lack-of-data uncertainty over the mixed-variable design space that consists of qualitative and quantitative material design variables. The design of electrically insulating nanocomposites is cast as a multicriteria optimization problem with the goal of maximizing dielectric breakdown strength while minimizing dielectric permittivity and dielectric loss. Within tens of simulations, this method identifies a diverse set of designs indicating the tradeoff between dielectric properties. These findings project data centric design, effectively integrating experimental data with simulations for Bayesian Optimization, as an effective approach for design of engineered material systems.