Featureless Optimization of Material Properties for Small Data Sets in Complex Ceramics
Electronic materials that exhibit phase transitions between metastable states (e.g., metal-insulator transition materials with abrupt electrical resistivity transformations) are challenging to decode. For these materials, conventional machine learning methods display limited predictive capability due to data scarcity and the absence of features that impede model training. In this work, we developed an adaptive optimization engine that overcomes these limitations
James Rondinelli (Northwestern University)
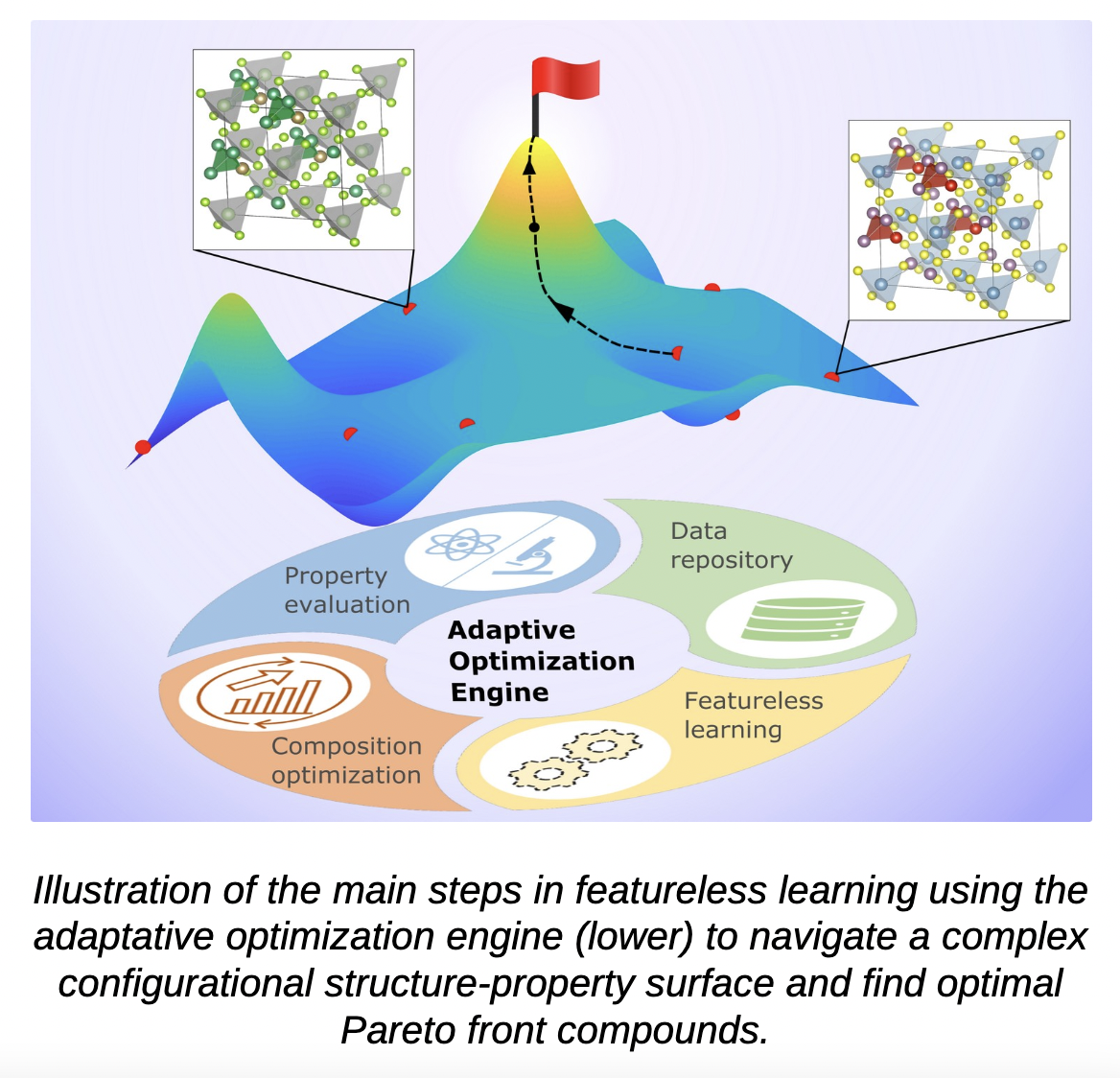
Electronic materials that exhibit phase transitions between metastable states (e.g., metal-insulator transition materials with abrupt electrical resistivity transformations) are challenging to decode. For these materials, conventional machine learning methods display limited predictive capability due to data scarcity and the absence of features that impede model training. In this work, we developed an adaptive optimization engine that overcomes these limitations. It:
- •Directly learns the composition-property relationship
- •Enables efficient co-design of functional materials
- •Suitable for limited data and descriptor availability
- •Applicable to inverse design problems
We used it to identify 12 functional and synthesizable metal-insulator transition (MIT) materials without a large starting data set or using hand-tuned features. These materials exhibit the lacunar spinel structure and cation ordered transition metals cations. The approach also led to identification of a semiconductor-to-insulator transition and improved understanding of the transport properties.