Learning Stability of AB2X6 Compounds to Guide Synthesis of Trirutile Oxides
We used machine learning and density functional theory (DFT) simulations to study crystal structure formation in the AB2X6 oxide and fluoride composition space.
James Rondinelli (Northwestern University)Stephen Wilson and Ram Seshadri (UCSB)
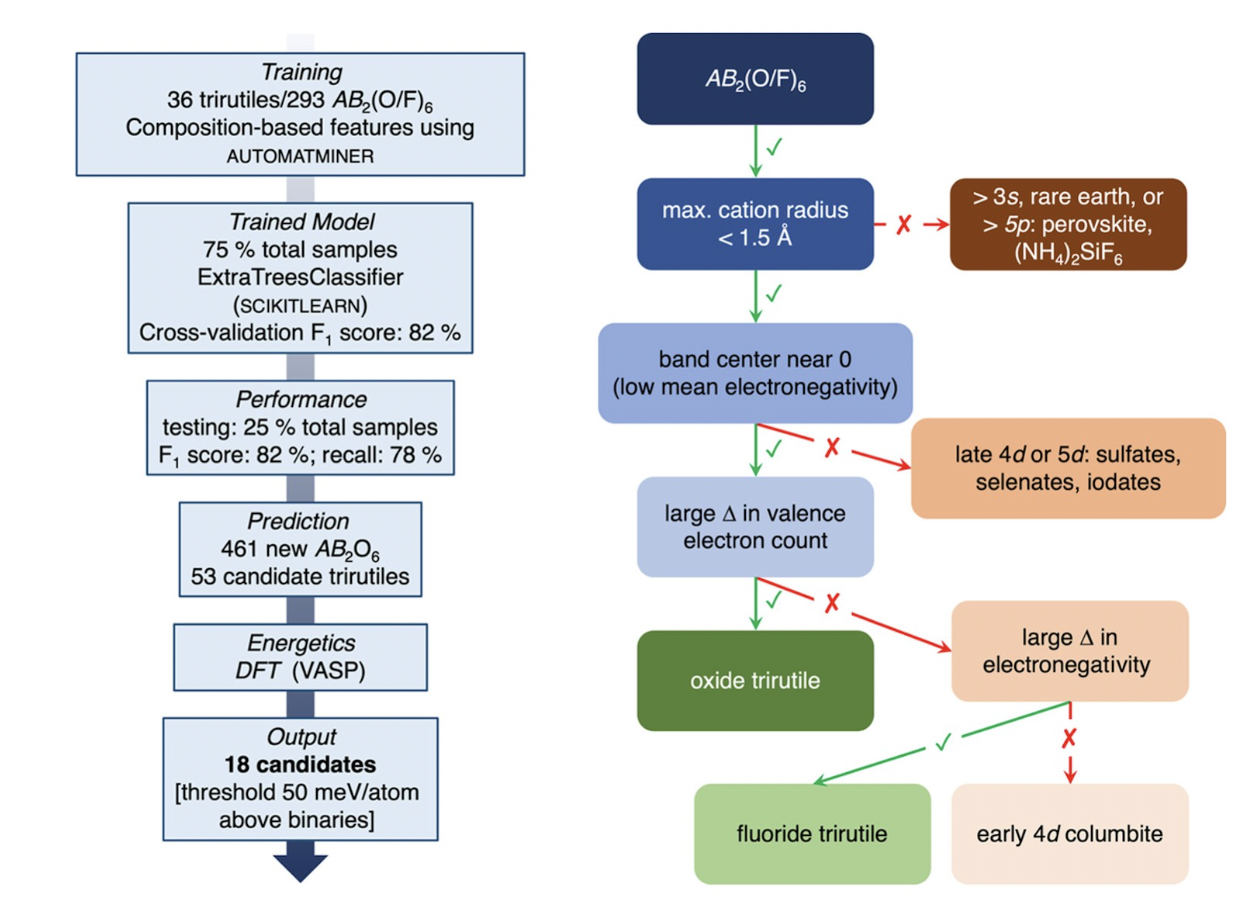
We used machine learning and density functional theory (DFT) simulations to study crystal structure formation in the AB2X6 oxide and fluoride composition space. We explored the underlying factors that determine whether an AB2X6 compound will crystallize in the trirutile structure with the goal of predicting new trirutile oxides. Through machine learning methods, we find that, consistent with factors determining crystallization in other structural families, geometric and bonding constraints are the most important features determining the formation of a trirutile structure. The trirutile structure is preferred over others when both the A and B atoms are relatively small and less electronegative.
Starting from 461 AB2O6 compositions, we predict 53 new candidate trirutile materials via machine learning. From DFT calculations, we find 18 of the 53 have a formation energy in the trirutile structure that is less than 50 meV/atom above the formation energy of the constituent binary oxides. From these 18 compounds, we prepared two novel AB2O6 compounds, TiTa2O6 and CrSb2O6, and find that they form, but as disordered rutiles under the conditions employed, rather than in an ordered trirutile structure.