Integrating Physics-based Models with Data-driven Methods for Materials Discovery
Metal-insulator transition (MIT) compounds are materials that can undergo an electronic phase changes and are promising platforms to build next-generation low-power microelectronics. Accelerated discovery is challenging using high-throughput screening because high-fidelity quantum-mechanical simulations are computationally prohibitive to perform.
James Rondinelli (Northwestern University)
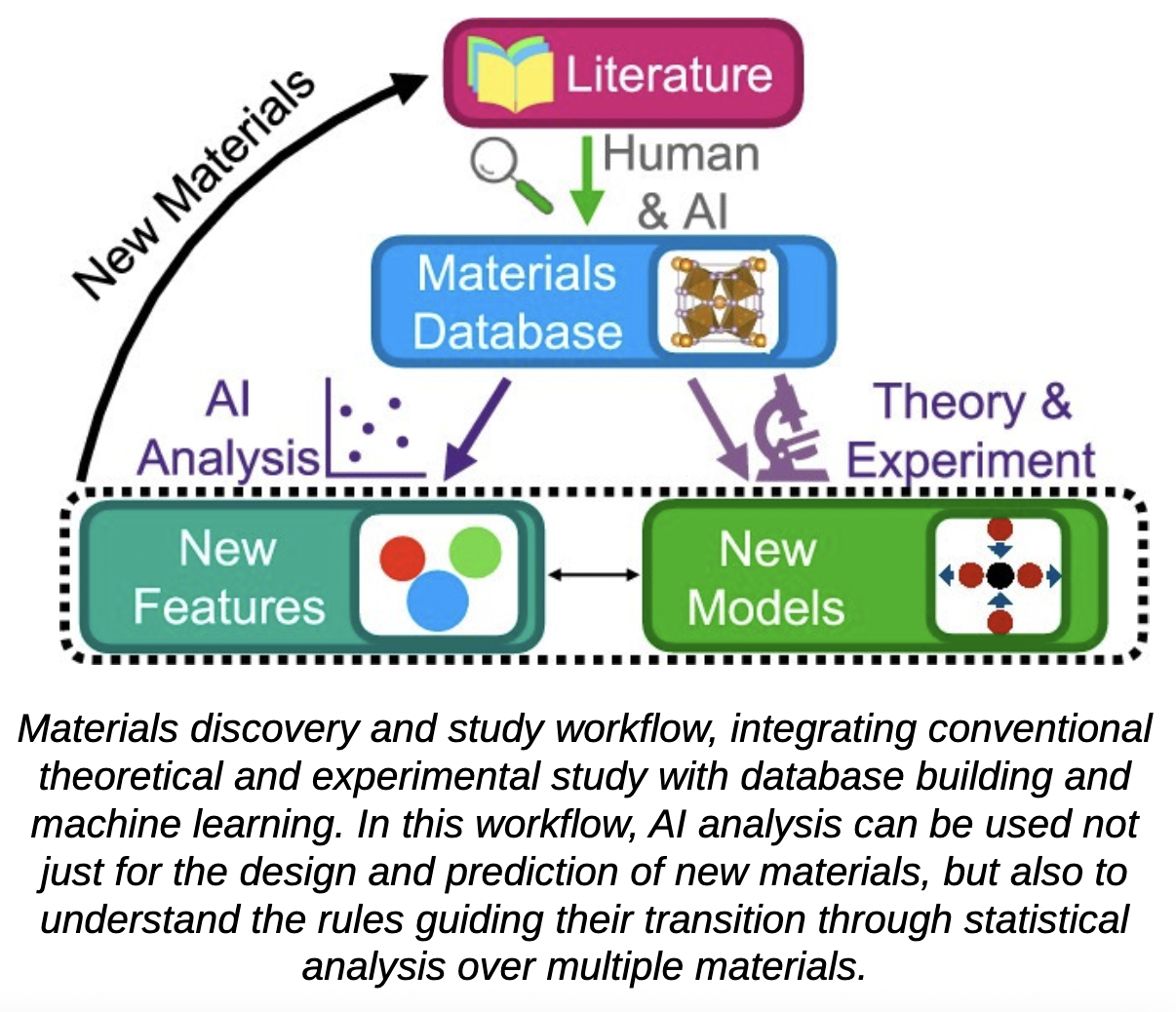
Metal-insulator transition (MIT) compounds are materials that can undergo an electronic phase changes and are promising platforms to build next-generation low-power microelectronics. Accelerated discovery is challenging using high-throughput screening because high-fidelity quantum-mechanical simulations are computationally prohibitive to perform. We solved this problem by building a supervised machine-learning model that can classify whether a material, given its structure as input, would exhibit a thermal MIT.
- •We created the first the public dataset of thermally-driven MIT compounds using natural language processing schemes in collaboration with the Olivetti group (at MIT) and publicly disseminated the new database.
- •Compounds were featurized using new physics-based descriptors, which were integrated into the matminer Python library for data mining the properties of materials.
- •We also deployed an easy-to-use online pipeline that can enable quick probabilistic of any crystalline material. Promising compounds identified with classification model are undergoing experimental validation, in collaboration with the Wilson group at UCSB.