Machine-learning Spectral Indicators of Topology
Topological materials are promising for next-generation energy and information applications. However, the experimental determination of topology can be painstaking, with a few limitations such as limited sample types, high technical barriers, and limited sample environment.
Mingda Li, Massachusetts Institute of Technology
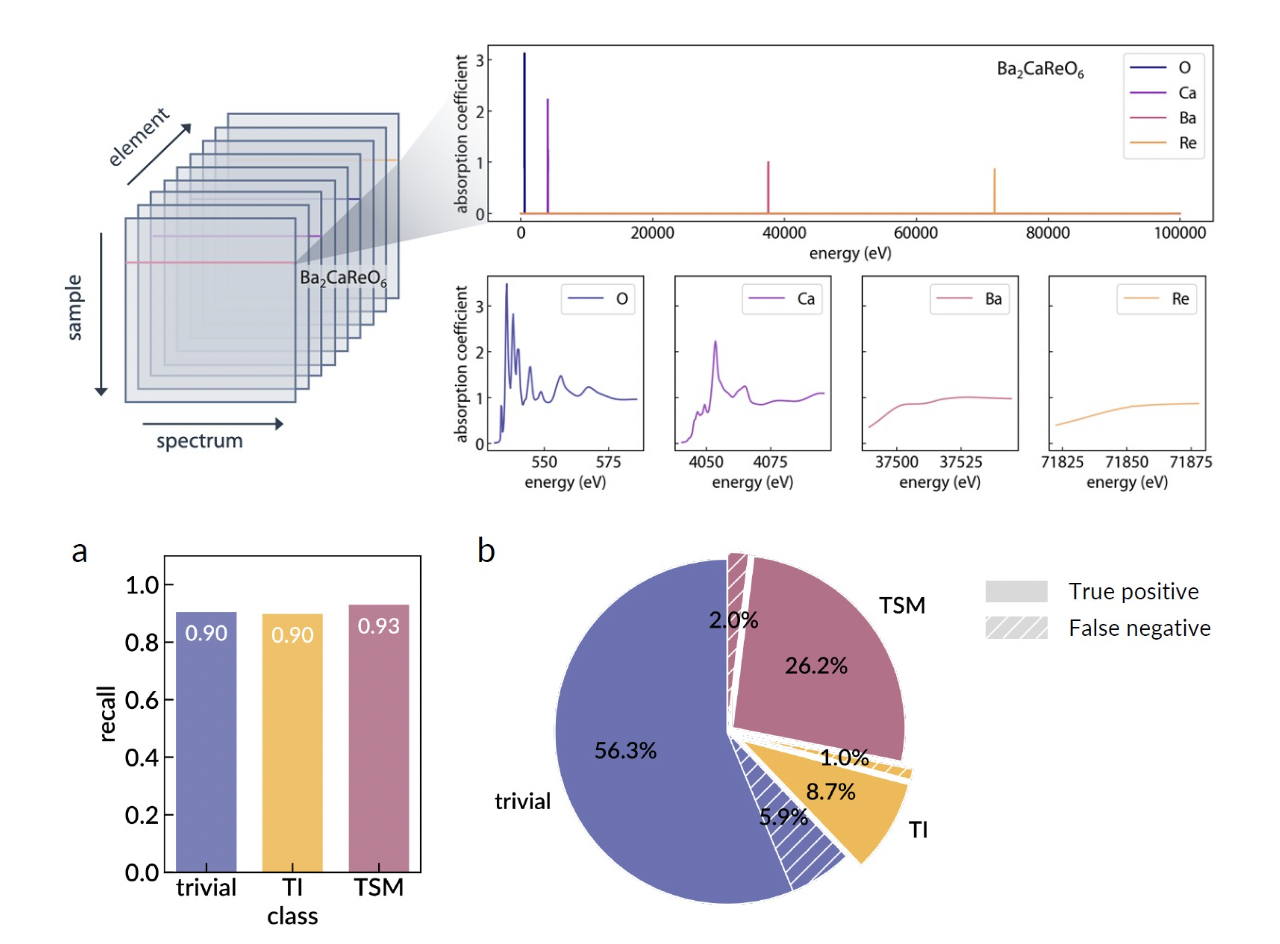
Topological materials are promising for next-generation energy and information applications. However, the experimental determination of topology can be painstaking, with a few limitations such as limited sample types, high technical barriers, and limited sample environment.
In this work, by designing a machine learning architecture to analyze simple X-ray absorption spectra, materials’ topological class can be determined with over 90% accuracy. This enables the topological determination of new topological materials with much reduced technical barriers in broader types of material candidates, and facilitates study of other phenomena like topological phase transition and amorphous topological materials.